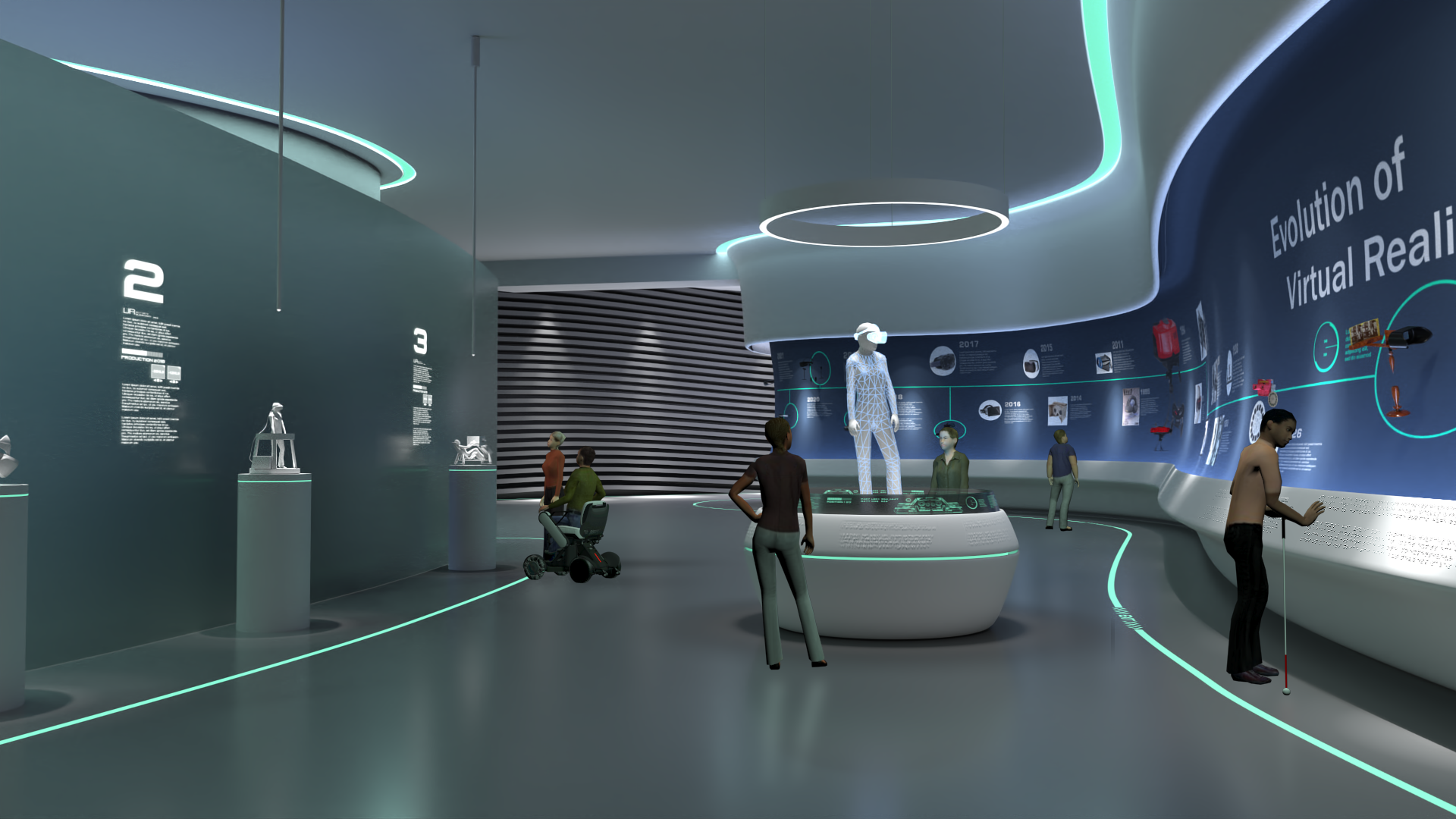
- using Gestures in 3-space
Annotated Bibliography
COPY currently shown bibliography
Tan, P., Han, X., Zou, Y., Qu, X., Xue, J., Li, T., Wang, Y., Luo, R., Cui, X., Xi, Y., Wu, L., Xue, B., Luo, D., Fan, Y., Chen, X., Li, Z., Wang, Z. L., Self-Powered Gesture Recognition Wristband Enabled by Machine Learning for Full Keyboard and Multicommand Input. Adv. Mater.2022, 34, 2200793. https://doi.org/10.1002/adma.202200793
- This study created a gesture recognition wristband to serve as a lightweight alternative to existing VR control systems that are more physically cumbersome. They achieved 92.6% accuracy and found the technology to have great potential.
Z. Lu, S. Cai, B. Chen, Z. Liu, L. Guo and L. Yao, "Wearable Real-Time Gesture Recognition Scheme Based on A-Mode Ultrasound," in IEEE Transactions on Neural Systems and Rehabilitation Engineering, vol. 30, pp. 2623-2629, 2022, doi: 10.1109/TNSRE.2022.3205026. Accessed at: https://ieeexplore.ieee.org/abstract/document/9881558
- This study tested a portable gesture recognition system using ultrasound with AI that can be used offline, finding it has a 96.92% accuracy rate, showing the technology has great potential for use in gesture-based interfaces in many contexts.
Fernández-Vázquez, D., Cano-de-la-Cuerda, R., & Navarro-López, V. (2022). Haptic Glove Systems in Combination with Semi-Immersive Virtual Reality for Upper Extremity Motor Rehabilitation after Stroke: A Systematic Review and Meta-Analysis. International Journal of Environmental Research and Public Health, 19(16), 10378.
- "The effectiveness of the virtual reality (VR) for the upper extremity (UE) motor rehabilitation after stroke has been widely studied. However, the effectiveness of the combination between rehabilitation gloves and semi-immersive VR (SVR) compared to conventional treatment has not yet been studied...The combined use of rehabilitation haptic gloves and SVR with conventional rehabilitation produces significant improvements with respect to conventional rehabilitation treatment alone in terms of functionality of the UE in stroke patients." (Fernández-Vázquez, D. et al. 2022)
Xing, T., Yang, Q., Jiang, Z., Fu, X., Wang, J., Wu, C. Q., & Chen, X. (2022). WiFine: Real-time Gesture Recognition Using Wi-Fi with Edge Intelligence. ACM Transactions on Sensor Networks (TOSN) [cited 2022 Sep 29] Available from: https://dl.acm.org/doi/pdf/10.1145/3532094
- "In this paper, we propose a lightweight Wi-Fi gesture recognition system, referred to as WiFine, which is designed and implemented for deployment on low-end edge devices without the use of any additional high-performance services in the process. Extensive experimental results show that the proposed system achieves fast recognition of various actions with an accuracy up to 96.03% in 0.19 seconds."