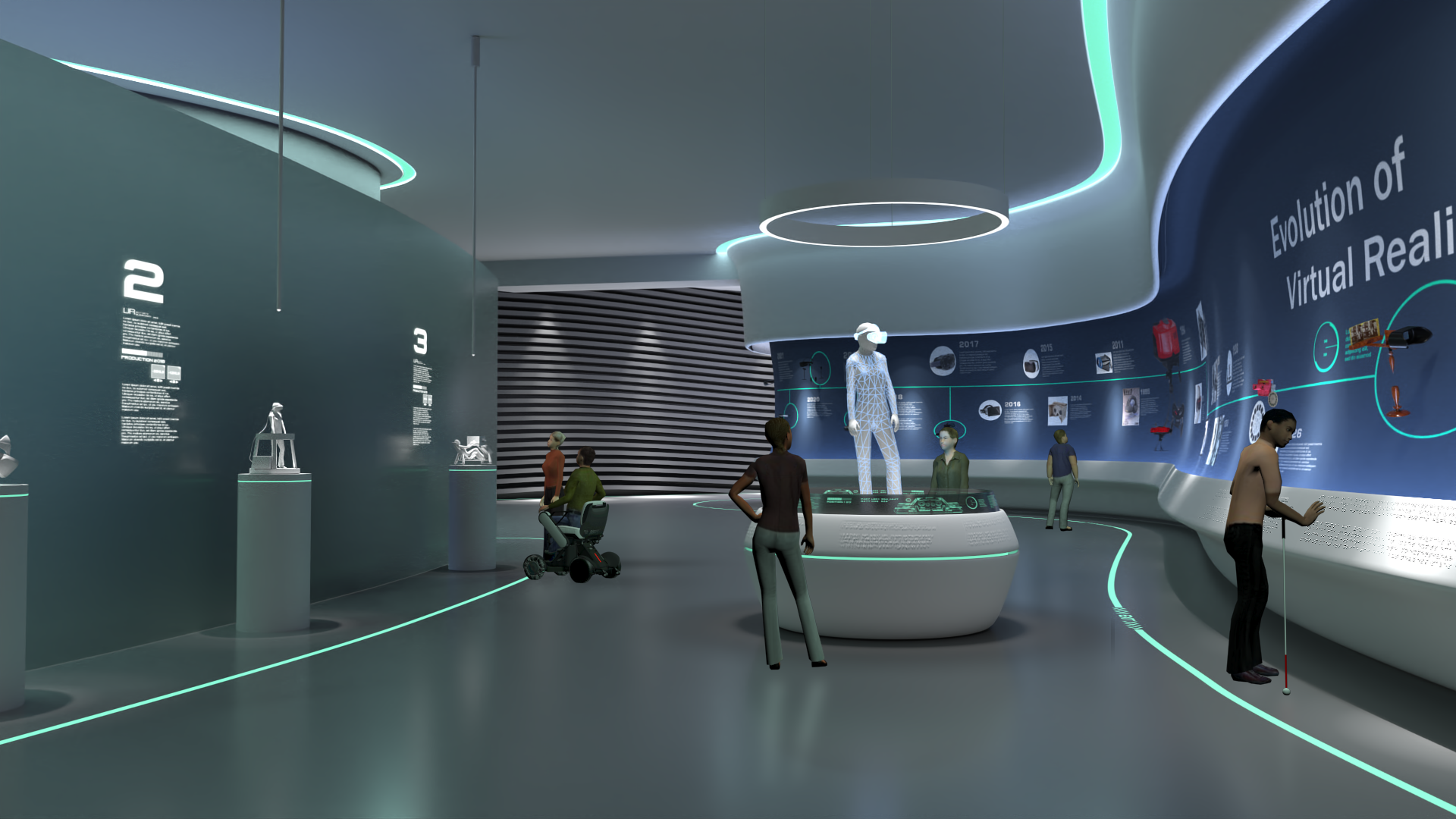
- to Enhance Input
Annotated Bibliography
COPY currently shown bibliography
Bragg, D., Koller, O., Bellard, M., Berke, L., Boudreault, P., Braffort, A., Caselli, N., Huenerfauth, M., Kacorri, H., Verhoef, T., Vogler, C., & Ringel Morris, M. (2019). Sign language recognition, generation, and translation. The 21st International ACM SIGACCESS Conference on Computers and Accessibility. https://doi.org/10.1145/3308561.3353774
- This paper presents the results of an interdisciplinary 2-day workshop, providing background to what is often overlooked by computer scientists, a review of the state-of-the-art, a set of pressing challenges, and a call to action for the research community.
Hameed, H., Usman, M., Tahir, A. et al. Pushing the limits of remote RF sensing by reading lips under the face mask. Nat Commun 13, 5168 (2022).
https://doi.org/10.1038/s41467-022-32231-1
- This paper aims to solve the fundamental limitations of camera-based systems by proposing a radio frequency (RF) based Lip-reading framework, having an ability to read lips under face masks. Through their research, these technologies might transcend their limitations of occlusion and ambient lighting with serious privacy concerns, and those limitations that arise in a coronavirus (COVID-19) environment
https://www.nvidia.com/en-us/studio/canvas/
NVIDIA Canvas is an AI-powered app that enables artists and designers to quickly create photorealistic images from rough sketches and doodles. It uses machine learning algorithms to turn rough sketches into digital artworks and supports various styles, including oil painting, watercolor, and pencil sketches. When it comes to generating Assistive Technology (AT) friendly layouts, Nvidia Canvas can help by allowing designers to create high-quality, visually appealing images that can be easily understood by AT users.
Tortora, S., Ghidoni, S., Chisari, C., Micera, S., & Artoni, F. (2020). Deep learning-based BCI for gait decoding from EEG with LSTM recurrent neural network. Journal of neural engineering, 17(4), 046011. Available from: https://iopscience.iop.org/article/10.1088/1741-2552/ab9842/pdf
- "Mobile Brain/Body Imaging (MoBI) frameworks allowed the research community to find evidence of cortical involvement at walking initiation and during locomotion. However, the decoding of gait patterns from brain signals remains an open challenge. The aim of this work is to propose and validate a deep learning model to decode gait phases from Electroenchephalography (EEG). Our results support for the first time the use of a memory-based deep learning classifier to decode walking activity from non-invasive brain recordings."